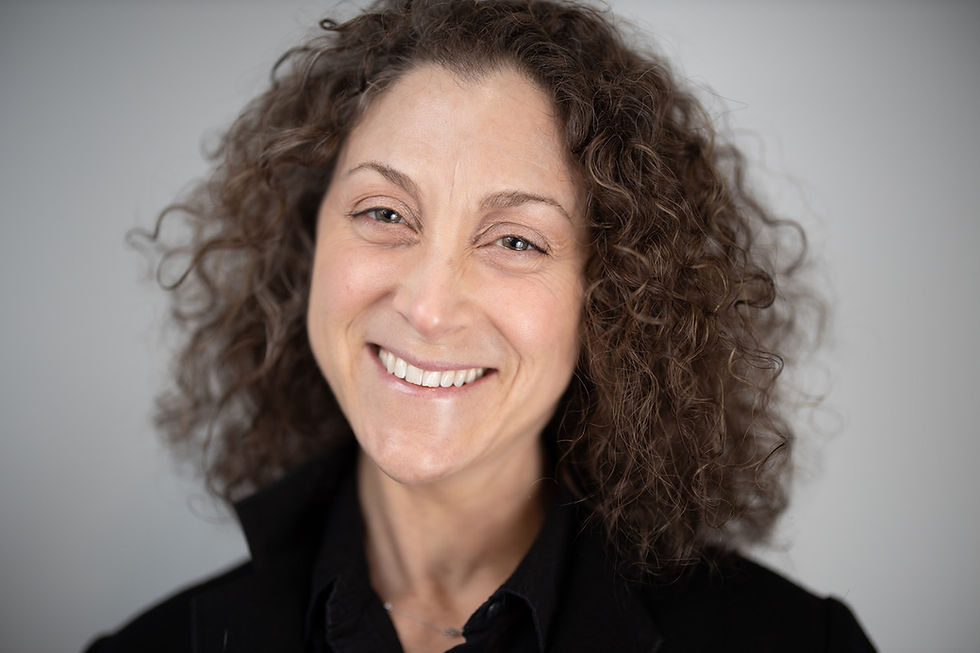
Rochelle Tractenberg is a tenured professor in the Department of Neurology, with appointments in Biostatistics, Bioinformatics & Biomathematics and Rehabilitation Medicine, at Georgetown University. She is a multi-disciplinary research methodologist and ASA-accredited Professional Statistician (PStat®), as well as a cognitive scientist focused on higher education curriculum design and evaluation. Her clinical and translational work integrates theories and principles of statistics, psychometrics, and domain-specific measurement to problems of assessment and the determination of changes in cognition, brain aging, and other difficult-to-measure constructs, using qualitative and quantitative methods. She is also an internationally recognized expert on ethical statistics and data science practice, having published two books, Ethical Practice of Statistics and Data Science and Ethical Reasoning for a Data-Centered World, in 2022. In addition to ethical statistics and data science practice, she has also contributed to guidelines for ethical mathematical practice (US based) and particularly, on how to integrate ethical content into quantitative courses. Professor Tractenberg is an elected Fellow of the American Statistical Association and of the American Association for the Advancement of Science, and was nominated for the 2022 Einstein Foundation Award for Promoting Quality in Research. Each of these nominations highlighted her commitment to, and support for, scientific stewardship.
Why did you decide to go into Statistics/Statistics Education?
Well! I went "into Statistics" kind of on a dare: I was always very bad at anything quantitative - math, chemistry, physics - which was deeply problematic during undergrad since I was pre-med (and one must *pass* calculus, chem, organic chem, and physics to get into med school -or did in the last century when I was in college). I double majored in Logic and Biopsychology, and did OK in the Boolean Calculus and experimental design courses, but abysmally in the pre-med core quantitative courses. I went to grad school and they noted that I might not be sufficiently ... prepared for the quantitative nature of the program, so I had to take a three-course calculus series. I literally did nothing but read and study calculus for an entire summer. That time, and the focus, helped me to realize I had been relying on my memory, and not developing problem solving skills, in all my earlier quantitative courses. The grad program was in Cognitive Science, and turned out to be very quantitative (like psychometrics, but not applied at all). I designed my thesis experiments and did all the analyses, but they were simply ANOVA and t-tests, with multiple corrections. In this program I learned how critical study design is for interpretable statistics, and (because it was Cognitive Science), I learned a great deal about learning and how instructors can (and some absolutely do not) facilitate learning. I also learned that people in general, and my students in particular, really do not want to learn statistics, but it is critical (and usually required!). My first job after that PhD was as a staff biostatistician for the national Alzheimer's Disease Cooperative Study - I consider that to have been a 5-year postdoc where I really learned an incredible amount about statistics, clinical trial design and analysis, but also how critically dependent on the instruments, and assumptions about measurement, modern clinical trials are. I completed a Master's in Public Health (MPH) with an emphasis in biometry and biostatistics. Measurement is an integral part of k-12 education, but the way we assess student learning in college and grad school is ...haphazard at best. When I left the biostatistician job for a faculty job at Georgetown, I had a lot of ideas about statistics education: it is critical for undergrad, grad, and medical students; it is essential that it be taught well and effectively; it is a topic that is typically reviled and most instructors don't love teaching students who are basically being forced to take this course; and it is generally taught without any consideration of modern cognitive and educational theory. Eventually I got a 5-year training grant, wherein I would learn about latent variable modeling. The program was in a college of education, so all the homework involved large datasets from k-12 assessments. I did all the homework twice; once with the designated data and again with either my own data from graduate students in the intro to biostats course I taught, or, with data from my biostatistician job. I ended up taking three courses that I hadn't planned to take, and with those three I earned a 2nd PhD - this one in Measurement, Statistics, and Evaluation. I also earned a Doctoral-level Certificate in Gerontology. All of these experiences reinforced my decision to go "into Statistics", but have also informed my work "in Statistics Education". One way my two PhDs and my work experience as a researcher are integrated is a curriculum development and evaluation tool I developed called a Mastery Rubric (https://masteryrubric.org/). I used that construct to create a curriculum map for statistical literacy (https://www.mdpi.com/2227-7102/7/1/3), and there is a new one in development for statistics and data science. I continue to work as a research methodologist, and to be concerned about how people who are experts in an area that is important for, but somehow inaccessible to, or apparently unwelcoming of, non-experts can "do better" at communicating the core concepts of their expertise. I just finished a white paper (June 2023) that integrates what is known about cognitive complexity into a communication model called "Diffusion of Innovation", originally published by Everett Rogers in 1965 (https://osf.io/preprints/socarxiv/f3ujn/). I hope that I can leverage my training and experience to help instructors in SDS at all levels to recognize how they can make statistics, data science, and quantitative reasoning more accessible to people who can easily feel discouraged from engaging with topics that might be hard for them at first. My experiences in college taught me that failures to "get it" should be avoided. I am stubborn, I guess, and persisted in the pre-med path in spite of really not "getting it" at all in the quantitative courses. I loved science and neuroscience in particular. I had to add the Logic major (leading to a B. A. degree) in order to graduate from the Biopsychology major (leading to a B. S. degree) with a B average! When I have had good statistics instructors, the courses have been inspirational! I want to help all students to have experiences like that.
What's a class/workshop at your workplace/university that you wish you could take and why?
I am interested in going through the MS program for data science at my institution - because it's free (for me). There is a lot of confusion about the definition of "data science", but one thing I am *really* not good at is computing. It's possible that I am not good at it because I've never had a good teacher, but also probably because I generally work with "small" data. I design clinical trials and my data analysis is very typically limited to hypothesis driven work, not data-driven work. I do think a lot about how to appropriately analyze human microbiomes (a complex ecosystem of microbes that inhabit skin (skin microbiome), gut, and other systems), so this could be a useful set of skills for me to learn! I might do this on my next sabbatical. :D
What Statistics Topic do you think is the most difficult to teach well?
It depends! I don't work with students until they're in a Master's or PhD program (although more typically they're already practicing physicians when I get them), and it is very difficult for people to unlearn terrible ideas they have about data and statistics. For example, Likert ratings are self-reports people give like, "strongly agree" or "neutral". If you have a 5-level Likert scale, the response range is "strongly agree", "agree", "neutral", "disagree", "strongly disagree". A typical way these ratings are entered into analysis is to assign each rating a number. Firstly, the number is totally arbitrary - do you assign "neutral" a zero, so that ratings range from -2 to 2? Or does the first option appear as a 1, and the ratings go from 1-5? But most importantly, assigning a number to the rating makes it appear like a) "strongly agree" and "agree" are equidistant from each other with "neutral" and "disagree"; and b) everyone's "agree" is the exact same level of agreement. In fact, "strongly agree" and "agree" are more similar to each other ("closer") than "agree" and "neutral". A person is much more likely to randomly choose between "strongly agree" and "agree" than they are to choose between "agree" and "neutral". Subjective ratings are incredibly variable, even when people give the same rating. But, the assignment of numbers to the ratings obfuscates this variability and gives a false impression of exchangeability of responses. I guess that means, my answer to this question isn't what I think is difficult to teach well but rather, one thing that might not get enough attention in a statistics or data science course is the fact that, just because your output says one thing, you really need to confirm your assumptions are met, and carefully consider alternative explanations to the results you observe. These ideas (a need for a positivist attitude) are difficult to teach well because of pressures to publish - apparently necessitating a "statistically significant result".
What advice would you give to someone who is new to teaching statistics?
1. While you need "right" and "wrong" answers in order to get scores and grades, it is important to encourage students to engage their verbal skills as well as their quantitative ones. Making sure that statistics and data science ideas become a natural part of thinking requires that we teach statistical thinking/reasoning, and not just the formulas or how to get the software to go.
2. It's ok to ask "why did you choose that (answer/method)?" or "what alternatives did you consider?" - these won't have right/wrong answers, but will challenge learners to justify their decisions. It will challenge the instructor to include this sort of information in their teaching, too (because you don't want to test on something you've never introduced!). Otherwise, the reason students choose methods might be due to the fact that they were the most recently presented in class - which of course will never work in the real world!
3. Carefully consider your learning outcomes when determining your assessments.
4. It is possible to integrate ethical reasoning, and considerations of ethical statistics and data science practice into your course! That can also satisfy some other learning outcomes for your course, program, or school, so it might be effort that is worth doing!
What is your go-to source for data?
Since my teaching is mostly limited to applied work nowadays, the data are already available to us from our research.
What statistics class(es) are you currently teaching? What statistics classes do you enjoy teaching the most?
I'm actually teaching (when I do) ethical reasoning and/or ethical statistical practice. This is typically a small course, and recently I'm only teaching short courses. But I really enjoy engaging with faculty, and learning about how they plan to integrate what we've done into their own courses.
What do you enjoy doing when you’re not working?
I do work like, almost all of the time. I sometimes have to force myself to take even a lunch break. I enjoy reading, particularly snuggled up with my two Rhodesian Ridgebacks - that never changed with covid! I used to enjoy traveling, that's only just getting started back up again with the World Statistics Congress (Ottawa) and JSM (Toronto) this year (2023). Next year I have work trips to Australia and Puerto Rico <so far> on the calendar. I always make sure to build in days for exploring the cities I visit. I love architecture, and always photograph manhole covers, doors, and the spectacular details of buildings in these cities. I also really like taking photographs of my pups and the natural world.
Kommentare